Since about 2013, and particularly over the last five years, off-the-shelf, commercial Machine Learning (ML) technology has enabled a not-so-quiet revolution in the oil and gas industry, transforming how hydrocarbons are located developed.
The development and applications of ML have occurred within overarching digital transformation initiatives that seek to transform energy companies and include Big Data analytics as another arrow in the digitalization quiver. ML is being applied to reveal greater details in the subsurface, identify potential hydrocarbon-bearing reservoirs, optimize well locations, and increase production. Complementing applications of ML, advances in automation, robotics, and drones are reducing accident and injury rates among workers in the field. And reductions in structural costs are close behind energy companies’ health, safety, and environmental objectives.
In 2019, Simon Flowers, the Chief Analyst and Chairman at Wood MacKenzie estimated that “U.S. $73 billion of potential annual savings for the industry is achievable in the next five years based purely on known technologies.”
Multi-CPU High-Performance Computing (HPC) has been used for decades for seismic data processing and advanced, computationally intensive oil and gas applications. ML accelerates seismic imaging and reservoir characterization by analyzing data using pattern-recognition methods. Here, tools for conducting rock stratigraphic analysis and fault detection on seismic data empower geoscientists with new ways of imaging the subsurface quickly while reducing drilling risks.
There are many subsurface features of interest to geoscientists, and ML enables investigations of all types of structures. Two such studies that are prime applications of ML include automatic Fault Detection and Stratigraphic Analysis. A fault occurs when two sides of rock have been displaced over time due to stress-induced natural movement in the rock. Faults pose drilling risks for hydrocarbons, present hazards for constructing wind turbines, pose challenges for Carbon Capture and Sequestration (CCS) reservoirs, and introduce risks for saltwater contamination of freshwater sources in coastal regions. The leading technique for Fault Detection uses Convolutional Neural Networks (CNN) for pattern recognition, operating on seismic data.
Stratigraphic Analysis, another application of ML to mapping subsurface features, is about understanding the distribution of strata through the earth and how rock layers interact. ML discriminates the lithofacies in strata, such as carbonates, shale, sandstone, siltstone, coal, and other depositional materials. Here, there are two broad classes of ML technologies: supervised and unsupervised. Supervised techniques employ pattern-recognition methods like CNN, where a geoscientist identifies a small region, and the algorithm identifies similar patterns in seismic data. This method is called “seismic facies classification.” Another more automated approach is Self-Organizing Maps (SOM), which classifies the region into user-defined patterns (“classes” or “neurons”) in the data without geoscientists giving the algorithm the “answer” up-front. SOM is an “unsupervised” machine learning method in the sense that the algorithm learns the data with little input from the user. Depending on the region’s geology, both approaches have valid applications and are often used together to enhance the results, a form of machine learning orchestration.
Figure 1, for example, shows seismic faults that have been identified using a combination of deep learning CNN and SOM. Geophysical Insights in Houston, Texas, for instance, was one of the early pioneers in producing commercial, off-the-shelf ML technologies for the energy industry. Energy companies are discovering more potential from ML technologies when multiple tools are applied in concert on the same problem, a process called “Machine Learning Orchestration.” The commercial ML market has seen many new entrants from technology and service firms since 2013 when Geophysical Insights launched their Paradise® AI workbench for seismic Analysis.
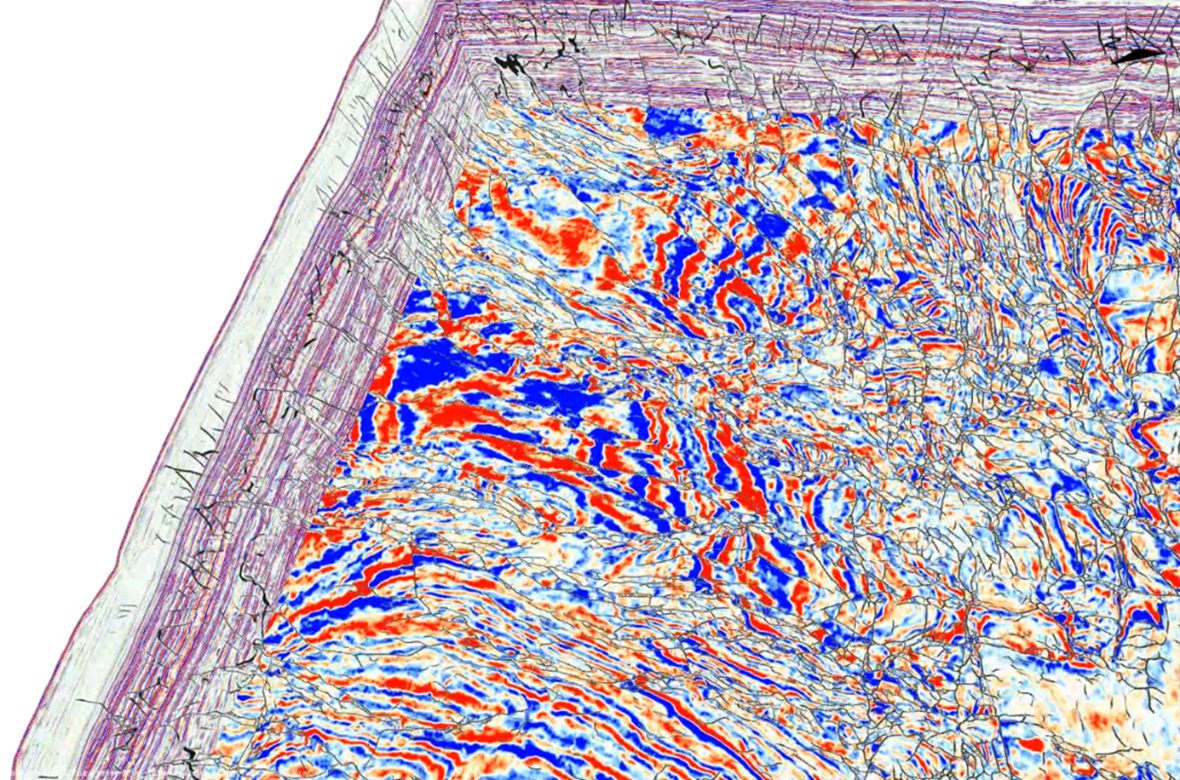
Figure 1: A Convolutional Neural Network (CNN) identifies faults (black lines), and a Self-Organizing Map (SOM) reveals different types of stratigraphic layers (colored regions). The two different ML applications can be used together to image subsurface conditions— source: Geophysical Insights, 2022.
Detecting seismic faults is also essential when locating candidate CCS reservoirs. Historically, geoscientists mapped seismic faults manually by visually inspecting 3-dimensional seismic reflection data and based on their understanding of a region’s geology. Faults that may connect a CO2 storage reservoir with a granitic rock basement at depth present leakage risks and have the possibility of inducing localized earthquakes (Hardage, 2022). Each injection of liquid Carbon Dioxide (CO2) into the CCS reservoir increases pore pressure in the reservoir rock, creating horizontal stress. Faults in a CCS reservoir that extend to the basement rock also allow the potential for seismicity that can travel 200-300 km from the storage site. Figure 2 shows a fault in seismic data; however, a large region will often include thousands of such faults. These risks increase the need to identify faults in a CCS reservoir as thoroughly as possible, and ML enables more rigorous and complete detection of faults than humans alone.
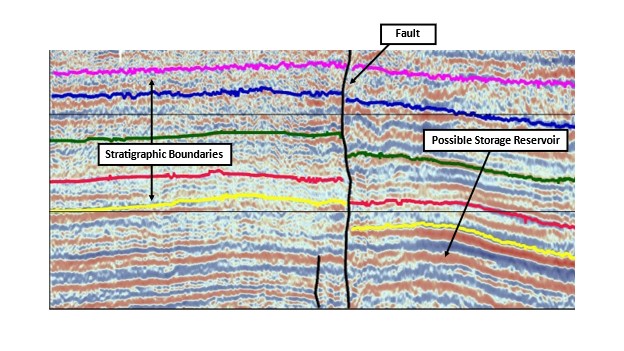
Figure 2: Figure 1. Seismic profile across a potential CO2 storage reservoir showing interpreted stratigraphic boundaries and faults. Source: Applying Machine Learning to Carbon Capture and Sequestration, The Science Times, 20 April 2022.
Another emerging application of ML-based Fault Detection and Stratigraphic Analysis is water management. Coastal water municipalities are concerned about freshwater contamination from seawater, threatening potable water sources, upsetting delicate environmental balances, and impacting local flora and fauna. Studies are underway using these new ML tools to help assess if deeper faults and fractures extend to the surface, allowing more seawater to migrate into freshwater sources. In the case of water wells near the coast, Fault Detection can indicate where a well crosses a fault, thereby introducing a risk of saltwater contamination of drinking water.
Judy Schulenberg, the President of Explorer Group 1 in Houston, Texas, is applying ML tools for Fault Detection and Stratigraphic Analysis to analyze coastal water sources. She shares this insight: “In addition to applications in the oil and gas industry, we have projects involving municipal water districts where saltwater infiltration contaminates freshwater wells. Many of these karsted carbonate environments are highly faulted. ML-based Fault Detection allows us to map these fault systems and apply seismic attributes to predict where new water wells should be drilled and whether older, less productive wells can be used for saltwater disposal. It’s a time and money saver but, more importantly, it’s an unbiased tool in our toolbox.”